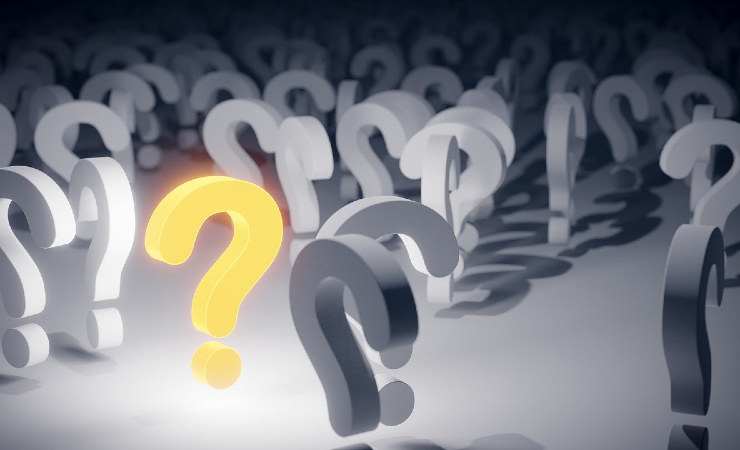
What are the critical issues AI raises for the life insurance and financial services industries? How is DEI changing the industry? During a recent online presentation, I addressed the top questions.
How should we define diversity, equity, and inclusion, and what are some important ways that DEI is changing our industry?
DEI is more than just numbers, so let’s look at some numbers, and what they do and don’t mean. According to the Bureau of Labor Statistics, the insurance industry in aggregate is diverse in employment: Women 59%, White 78%, African American 13%, Asian 6%, and Hispanic or Latino 12% (the numbers don’t add up to 100%, because there is overlap in the categories). Compared to the overall population, the diversity seems to be reasonable. Women are over-represented, White, African American, and Asian are about even but Hispanic/Latino is underrepresented by almost 50%.
Behind the numbers, minority groups are not benefiting from the proportion of women who are predominantly white. Even more important, the numbers do not portray the difference in distribution of low paid to high paid positions.
In other words, these numbers may seem respectable on the face of it, but you cannot speculate how diverse decision-making roles are. A popular notion is that diversity in the groups responsible for AI, underwriting, claims and even marketing and distribution lead to better outcomes, but it’s not likely that transition is very mature. So, the conclusion is diversity metrics are not an indicator of diversity in thinking and effects. Companies, not only insurance companies, must devise ways to increase diverse input into meaningful decision-making roles.
This may be a little provocative, but as AI and streamlined, automated processes WILL replace a lot of the work currently done by the lower-skilled employees, you must conceptualize how to upskill them and into more impactful roles.
The U.S. Supreme Court recently issued several high-profile decisions related to diversity and discrimination. In light of this, what are some ways that we, as insurers and as people, can make sure we’re still being inclusive?
It would be an understatement to say that DEI is a little different after the SCOTUS decision in 303 Creative v. Elenis. It is just the opening chapter in what will likely be years of litigation from people looking to push the limits around state and local laws restricting and providing civil rights protections for various minority and protected groups.
The Affirmative Action ruling is troubling too. Companies may second-guess their DEI initiatives having a chilling effect over corporate leaders who will cut back on DEI programs because of legal concerns involving recruitment, hiring, evaluations and promotions. The optics could be problematic but there are things that don’t involve HR decisions. Targeted outreach, auditing policies and procedures to remove implicit bias or creating employee resource groups, and mentorship These are not regulated by Title VII of the Civil Rights Law because they do not make explicit decisions about hiring or promoting.
How might insurance carriers need to change their internal operations or internal systems to better incorporate DEI considerations?
DEI initiatives have been proven to increase employee engagement and productivity, reduce absenteeism and turnover rates, improve customer service satisfaction levels, foster a more innovative culture, and create better problem-solving abilities among teams.
Better business performance – insurers face many new challenges and new, diverse skillsets are needed for success. Risk reduction is achieved by creating diversity of thought, resulting in less chance of groupthink and overall better risk management.
For the community, think about procurement. One method to enhance DEI outcomes is to explore alternative sourcing of goods and services. Supplier diversity procurement is the proactive and intentional inclusion of businesses owned by members of groups that have been historically marginalized in the economy, such as women, people of color, LGBTQIA+ people, and people with disabilities
What are some examples of pricing factors insurance companies use that we might need to reconsider?
Insurance is a complicated problem. Features like age, gender, location, etc. are a potentially negative classification in most practices. Still, for actuaries, a negative bias is a part of their business, and it’s a thin line separating analysis and discrimination. The use of credit scores in insurance flies in the face of DEI. One excuse I heard from an actuary was, “People with higher FICO scores manage money better than those with lower scores, which implies that those with lower FICO scores don’t manage other aspects of their lives.” I gasped when he said that. That is a type of bias that you won’t find directly in the data, just the results.
What can actuaries do to reduce bias in their models?
The world is structurally biased (there are inequities to the systemic disadvantage of one social group compared to other groups with whom they coexist) and that makes biased data. Observation is a process. When we create data, we choose what to look for.
Every automated system encodes a value judgment. Accepting training data as given implies structural bias does not appear in the data and replicating the data would be unethical.
Key conclusion: different value judgments can require contradictory fairness properties, each leading to different societal outcomes. Companies must document data collection processes, worldviews, and value assumptions. Value decisions must come from domain experts and affected populations; data scientists should listen to them to build values that lead to justice.
It’s a tricky question. Rating and reserving in Life and Health (and general personal lines, too) fundamentally rely on attributes that in other areas (lending, employment, even marketing) are now carefully being evaluated. So, one way to look at it is that AI is biased if (it) weights some factor as material in its prediction, when that factor is not genuinely predictive but is an artifact of its training dataset being distorted. This might be because a partial training dataset was selected in an unrepresentative way, or because there is a more general societal bias. We need to be able to give explanations for decisions made by black box models – this is a problem companies need to address
For a company just getting started on their DEI journey, how would you recommend they start?
It’s important for members of minority or protected groups to see people like them in the organization whose practices are admired. When I first started out as an actuary, my boss was an African American man who graduated from Fisk University, a historically Black University. It was unusual in those days, but he was a wise and kind mentor, and I learned early the value of working with diverse people and learning from their experience.
Senior execs need to be clear that they are behind DEI and express their reasons, as opposed to making gratuitous comments and disappearing. Frankly, not everyone in the organization may be onboard with the idea. Like my grandfather once told me: “Those who love us, love us. For those who don’t, may the Good Lord turn their hearts. But if their hearts can’t be turned, he should turn their ankle so we can recognize them by their limp.” If SOME are disruptive or openly hostile, HR should have a policy to deal with it. That goes for everyone, including those who in diverse groups.
What does the future hold for DEI?
Like Niels Bohr said, prediction is hard because it’s about the future. I don’t think a single company, or a single industry can solve the diversity and inclusion problem, but our demographics are already diverse and are becoming more so. The status quo is a horrible injustice.
Generative AI is a very hot topic this year. How might these new AI capabilities impact DEI efforts?
Honestly, we don’t really know. Like Judea Pearl said, it’s just curve-fitting, but that’s like saying a violin is just wood and cat gut and horsehair. ML and NLP under the covers are well-understood math – statistics, linear algebra, probability theory, and calculus/differential equations but it’s what they produce is what matters. Symbolic models are closer to intelligence but quite unfashionable now.
I could be wrong, but the mega LLM’s are already in jeopardy. The cost of training and inference is astronomical, and will remain concentrated with the few giant companies. They are already being sued for copyright violations. I believe the promise of the technology will be training models on your own curated data, and additional external curated data. This should limit the error rates and hallucinations, allowing the technology to be applied to useful tasks, augmenting skills and providing a path forward for those relieved of the tedious work that is not highly valued.
How do you determine fairness, and how can you measure fairness?
You can, and many have, devised mathematical models for fairness that are complete and consistent but in common use are hopelessly inadequate, I’d put disparate impact in that category because it makes the fundamental statistical error of taking ratios of ratios. Example: at one point Derek Jeter had a lower batting average than David Justice in two consecutive years, but if you aggregated the two years, you would see Jeter had the higher average. You can look that up in MLB statistics.
Any model is dependent on your definition of fair, and that’s a slippery slope. For our projects, we prefer a method for measuring fairness called “Group Benefit Equality.” Group benefit equality aims to measure the rate at which a particular event is predicted to occur by the model within a subgroup compared to the rate at which it happens. If our model predicts a subgroup will get the loan 60% of the time, but experience shows that only happens 45% of the time, the metric is .75, which is poor. This is an active area of research. It does however address the issue of causality – what features of the model drive certain outcomes. There are interesting applications from Game Theory, such as Shapley Numbers that measure how each variable affects the outcome. This is an incomplete analysis, but it’s a start.
End note: these answers are derived from an online presentation I did for the LIMRA/LOMA/SOA 2023 Supplemental Health, DI & LTC Conference LIMRA (Life Insurance Marketing and Research Association) & LOMA (Life Office Management Association) say that: “Our purpose is to advance the life insurance and financial services industry by empowering our members with knowledge, insights, connections, and solutions.” SOA (Society of Actuaries) “is dedicated to research in actuarial science, professional development and education, and professional standard.” I want to thank these organizations for inviting me to offer my thoughts, and especially to thank the organizers for their excellent preparation as I was not able to appear in person.